How to Train Stable Diffusion? Kodexo Labs Can Help Business Grow With Stable Diffusion
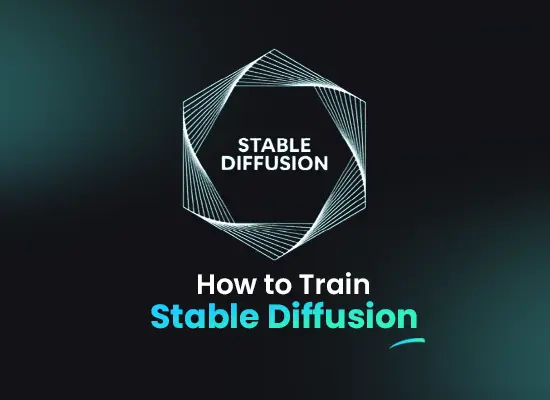
Want to take control and personalize the incredible image generation of Stable Diffusion? Dive into this guide to learn how to train your own Stable Diffusion model! We’ll explore the process, from gathering the right data to fine-tuning the model for your specific desires, empowering you to unleash the full potential of Stable Diffusion.
What is Stable Diffusion?
Stable Diffusion, released in 2022 by Stability AI, is a game-changer in the realm of generative AI. This powerful tool utilizes a deep learning approach, specifically diffusion models, to create high-quality images based on textual descriptions. Imagine feeding Stable Diffusion the prompt a majestic tiger prowling through a lush jungle and witnessing the AI conjure a photorealistic image that matches your vision! But how does this magic happen?
Stable Diffusion is pre-trained on a massive dataset of text and image pairs. This training allows it to understand the relationship between words and their visual representations. However, the beauty of Stable Diffusion lies in its ability to be further customized. Here’s where the concept of training Stable Diffusion comes in.
By providing the model with your own set of images and their corresponding descriptions, you can fine-tune Stable Diffusion to better understand your specific style or domain. This process, known as training stable diffusion with custom images or training a stable diffusion model, allows you to tailor the generated images to your preferences.
Training Stable Diffusion can be done in two main ways: from scratch or through transfer learning. Training stable diffusion from scratch involves feeding the model a massive dataset and letting it learn everything from the ground up. This approach requires significant computational resources and expertise.
A more accessible option is training stable diffusion locally through transfer learning. Here, you leverage the pre-trained knowledge of Stable Diffusion and refine it with your custom data. This method requires considerably less data and computational power, making it ideal for individual users.
Whether you choose to train Stable Diffusion from scratch or leverage transfer learning, the core concept remains the same: you’re shaping the model’s understanding to generate images that align with your vision. With its versatility and user-friendliness, Stable Diffusion opens doors for artists, designers, and anyone who wants to explore the exciting world of AI-powered image creation.
Seamless Collaboration | Cost-Efficient Solutions | Faster Time-to-Market
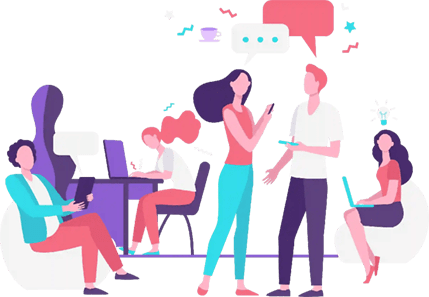
Training Stable Diffusion Models with Artificial Intelligence (AI):
Stable Diffusion has become a powerhouse in the realm of Artificial Intelligence (AI) and AI-powered image generation. But how do you train this model to create the specific visuals you desire? This guide explores seven crucial techniques to conquer the art of training Stable Diffusion models.
Whether you’re aiming to train a Stable Diffusion model entirely from scratch or customize it with your own unique image library, this breakdown offers valuable insights. We’ll delve into methods for both training stable diffusion from scratch and training stable diffusion with custom images. Here are 7 key approaches to consider when venturing into training Stable Diffusion models:
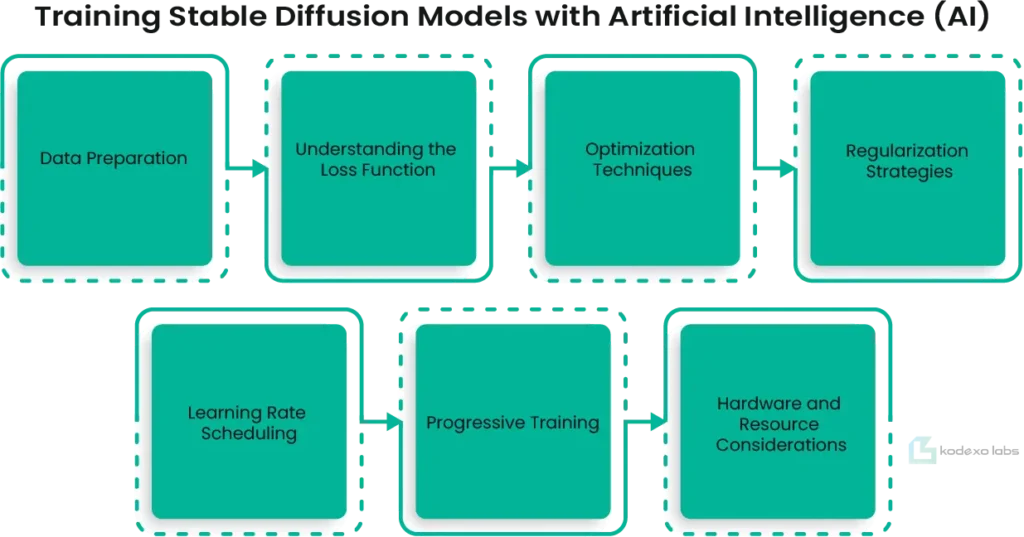
1- Data Preparation:
The foundation of any successful training process lies in well-curated data. Gather high-quality images relevant to the concepts you want your model to generate. This is especially important for training stable diffusion with custom images.
2- Understanding the Loss Function:
The loss function acts as a guide for the model, measuring the discrepancy between the generated image and the desired outcome. Choosing the appropriate loss function is vital for effective training.
3- Optimization Techniques:
Optimizers like Adam or SGD play a critical role in adjusting the model’s internal parameters during training. Selecting the right optimizer can significantly impact the convergence speed and overall quality of the trained model.
4- Regularization Strategies:
Regularization techniques help prevent overfitting, a situation where the model memorizes the training data too well and struggles with unseen examples. Techniques like weight decay and dropout can be implemented during training stable diffusion models.
5- Learning Rate Scheduling:
The learning rate controls how much the model’s parameters are updated in each iteration. Utilizing learning rate schedules ensures the training process remains stable and avoids getting stuck in local minima.
6- Progressive Training:
For complex tasks, consider a progressive training approach. This involves starting with a simpler model architecture and gradually increasing its complexity as training progresses. This can be particularly beneficial for training stable diffusion from scratch.
7- Hardware and Resource Considerations:
Training Stable Diffusion models can be computationally expensive. Explore options for leveraging cloud platforms or powerful GPUs for efficient training stable diffusion locally.
By mastering these techniques, you’ll be well-equipped to unlock the full potential of Stable Diffusion and train models that generate stunning and creative images based on your specific needs through AI development.
Perks to Training Stable Diffusion from Scratch:
While pre-trained Stable Diffusion models offer impressive capabilities, venturing into training your own model from scratch unlocks a treasure trove of advantages. Here’s a breakdown of the key benefits you can reap:
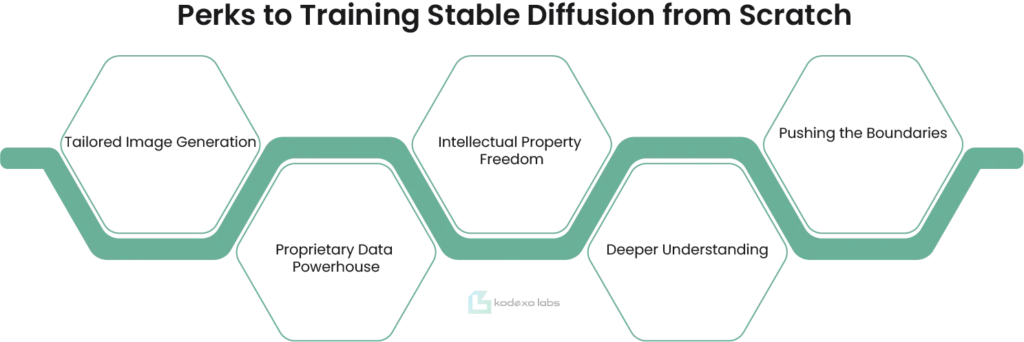
1- Tailored Image Generation:
Training a stable diffusion model with your custom dataset allows you to mold the model’s understanding towards a specific style or domain. Imagine training with nothing but cat pictures! You’ll be able to generate all sorts of feline imagery, from realistic breeds to fantastical cat creatures, all thanks to training stable diffusion with custom product development for custom images.
2- Proprietary Data Powerhouse:
Working with sensitive or confidential data? Pre-trained models might not be ideal. Training stable diffusion locally on your own machine with your proprietary data ensures complete control and privacy. This opens doors for applications in fields like medical imaging or product design.
3- Intellectual Property Freedom:
When you train a stable diffusion model from scratch, you own the entire process. This eliminates concerns about violating intellectual property rights associated with pre-trained models. You can freely use and commercially deploy your trained stable diffusion model without limitations.
4- Deeper Understanding:
The process of how to train Stable Diffusion from scratch fosters a deeper understanding of the model’s inner workings. You’ll gain valuable insights into how it interprets text prompts and translates them into visuals, empowering you to fine-tune the training process for even better results.
5- Pushing the Boundaries:
Contributing to the bleeding edge of AI research? Training from scratch allows for experimentation with different architectures and training parameters. This opens doors for pushing the boundaries of Stable Diffusion’s capabilities and potentially creating groundbreaking advancements in the field.
While the computational resources required for training a stable diffusion model from scratch can be significant, the potential benefits for specific applications and research endeavors make it a compelling option.
Is Training Stable Diffusion with Custom Images Easy?
Training Stable Diffusion with custom images can be a fascinating endeavor, allowing you to tailor the model’s output to your specific needs. However, the ease of this process depends on your technical background and desired level of control.
There are two main approaches: training a stable diffusion model from scratch and fine-tuning a pre-trained model with your custom images. Training a stable diffusion model from scratch is an incredibly complex task requiring significant computing power and expertise in Machine Learning development. This approach is generally not recommended for beginners due to the resource intensity and the vast amount of data required.
Fine-tuning a pre-trained model with your custom images, often referred to as training stable diffusion with custom images or using DreamBooth, is a more accessible option. Several online resources offer tutorials and tools to simplify this process. However, it still involves some technical knowledge and access to a powerful computer or cloud resources.
The complexity lies in preparing your custom image dataset, ensuring a consistent style and format. Additionally, tools like DreamBooth require some scripting knowledge to configure and run the training process.
While training stable diffusion locally on your personal computer is technically possible, it’s often impractical due to the aforementioned resource requirements. Cloud-based solutions are more realistic for most users.
In conclusion, although not a walk in the park, training stable diffusion with custom images offers a unique way to personalize the model’s output. By understanding the two main approaches – training from scratch and fine-tuning – and your own technical comfort level, you can determine the feasibility of this exciting project.
How to Train Stable Diffusion?
Stable Diffusion is a powerful deep learning model used for generating high-quality images from text prompts. To harness its full potential, users often seek methods on how to train Stable Diffusion to better suit their specific needs. Training Stable Diffusion involves various techniques, including the use of custom images, training locally, and even starting from scratch. This guide explores ten effective ways to train Stable Diffusion, ensuring that you can tailor the model to your unique requirements.
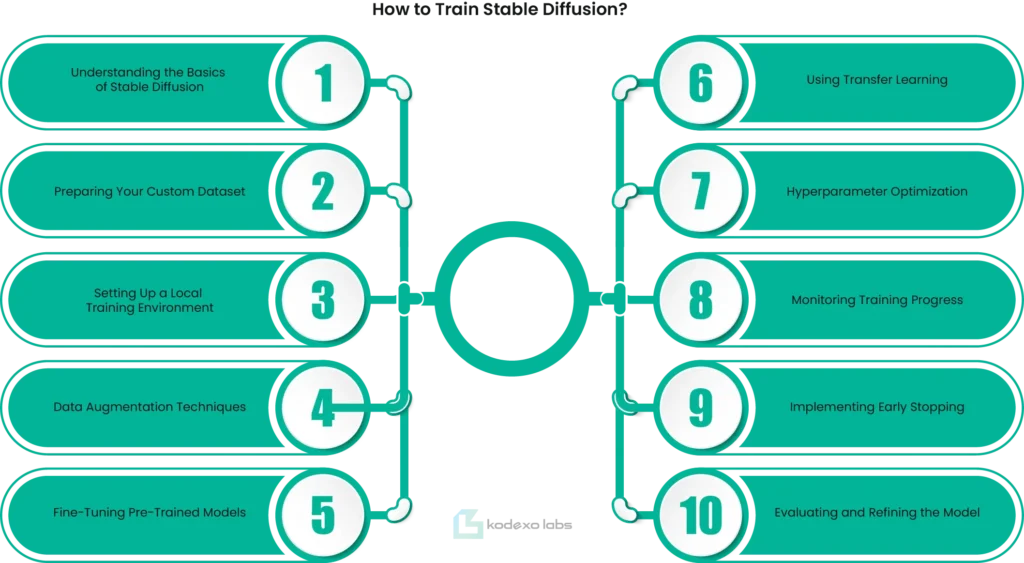
1- Understanding the Basics of Stable Diffusion:
Before diving into training Stable Diffusion, it is crucial to understand the underlying architecture and how it generates images from text. Familiarize yourself with the model’s structure and the role of diffusion processes.
2- Preparing Your Custom Dataset:
Training Stable Diffusion with custom images involves curating a high-quality dataset that reflects your desired output. Ensure that your images are diverse, well-labeled, and relevant to the tasks you want the model to perform.
3- Setting Up a Local Training Environment:
For those interested in training Stable Diffusion locally, setting up a robust environment is essential. Install necessary libraries such as PyTorch, TensorFlow, and other dependencies. A powerful GPU will significantly enhance the training speed.
4- Data Augmentation Techniques:
Enhance the diversity and quantity of your training data engineering services for data augmentation. Techniques such as rotation, scaling, and flipping can create varied versions of your custom images, enriching the training stable diffusion model process.
5- Fine-Tuning Pre-Trained Models:
Rather than training Stable Diffusion from scratch, consider fine-tuning a pre-trained model. This approach saves time and computational resources while leveraging the strengths of existing models.
6- Using Transfer Learning:
Transfer learning is an effective method for training Stable Diffusion with custom images. By adapting the pre-trained weights to your specific dataset, you can achieve high-quality results with less training data.
7- Hyperparameter Optimization:
Tuning hyperparameters such as learning rate, batch size, and number of epochs is critical in the training process. Experiment with different configurations to find the optimal settings for your training stable diffusion model.
8- Monitoring Training Progress:
Keep track of the training metrics, including loss and accuracy. Use tools like TensorBoard to visualize the training process and make necessary adjustments in real-time.
9- Implementing Early Stopping:
To prevent overfitting, implement early stopping techniques. This method monitors the model’s performance on a validation set and halts training when improvements plateau.
10- Evaluating and Refining the Model:
After training stable diffusion locally or otherwise, evaluate the model using test data. Assess its performance in generating images and refine it further based on the evaluation results.
Training Stable Diffusion can significantly enhance its performance and adaptability to specific tasks. Whether you are training Stable Diffusion with custom images or training Stable Diffusion from scratch, the techniques outlined above provide a comprehensive guide to optimize your efforts. By following these steps, you can achieve a highly customized and efficient stable diffusion model tailored to your unique needs.
What are the Benefits of Training Stable Diffusion Locally?
Training Stable Diffusion models locally offers a myriad of benefits for developers and researchers looking to harness the power of AI and machine learning. By understanding how to train Stable Diffusion models, especially with custom images and from scratch, one can achieve significant advantages in terms of control, customization, and cost efficiency. Here, we explore the nine key benefits of training Stable Diffusion locally, providing insights into why this approach is increasingly popular.
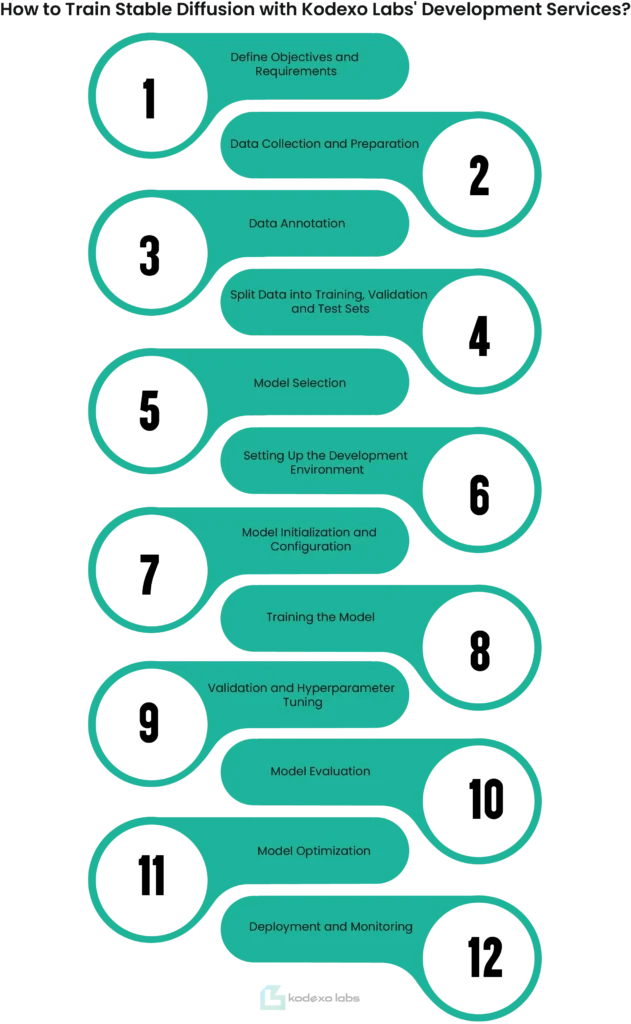
1- Full Control Over Data:
Training Stable Diffusion locally allows users complete control over their data. When training Stable Diffusion with custom images, you can ensure that the data used is relevant and accurate. This control is crucial for sensitive projects where data privacy is paramount.
2- Enhanced Customization:
One of the standout benefits of training Stable Diffusion locally is the ability to tailor the model to specific needs. Whether you are training Stable Diffusion from scratch or enhancing an existing model, local training facilitates fine-tuning and tweaking according to unique project requirements.
3- Improved Data Privacy and Security:
Training Stable Diffusion models locally significantly enhances data privacy and security. By keeping all processes in-house, organizations can prevent data leaks and ensure compliance with privacy regulations. This is especially important when training Stable Diffusion with custom images that might contain sensitive information.
4- Cost Efficiency:
Training Stable Diffusion locally can be more cost-effective in the long run. While initial setup costs might be higher, local training avoids the recurring costs associated with cloud services. This is particularly beneficial for large-scale projects where continuous training is required.
5- Better Performance Optimization:
Local training provides the opportunity to optimize performance according to the available hardware. When training Stable Diffusion models locally, developers can leverage specific hardware configurations to enhance computational efficiency and reduce training times.
6- Accessibility and Flexibility:
Training Stable Diffusion models locally offers greater accessibility and flexibility. Developers can work on models without the need for a constant internet connection, making it easier to continue work in various environments. This flexibility is invaluable for iterative development processes.
7- Independence from Cloud Services:
By training Stable Diffusion locally, organizations become less dependent on third-party cloud services. This independence can reduce the risks associated with service outages, data breaches, and vendor lock-in, providing more robust and reliable model training operations.
8- Scalability:
Training Stable Diffusion from scratch locally allows for scalability tailored to specific project needs. As project requirements grow, local infrastructure can be scaled up accordingly, providing a seamless expansion path without the limitations often imposed by external service providers.
9- Enhanced Learning and Skill Development:
For developers and researchers, training Stable Diffusion models locally offers a deeper understanding of the underlying processes. Engaging directly with the training setup enhances learning and skill development, fostering a more profound expertise in model training and AI development.
In conclusion, understanding how to train Stable Diffusion locally brings numerous benefits, ranging from enhanced control and customization to cost efficiency and improved security. Whether you are training Stable Diffusion with custom images or embarking on training Stable Diffusion from scratch, local training offers unparalleled advantages that make it an attractive option for many AI and Machine Learning Operations (MLOps) projects.
Seamless Collaboration | Cost-Efficient Solutions | Faster Time-to-Market
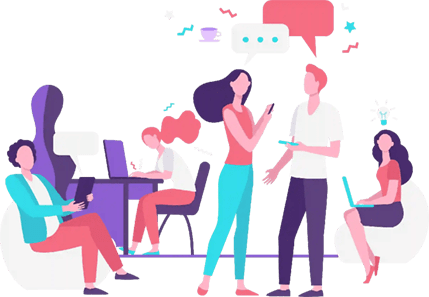
How to Train Stable Diffusion with Kodexo Labs' Development Services?
Training stable diffusion models, particularly for applications such as stable diffusion, requires a well-structured and systematic approach. Kodexo Labs, renowned for its advanced development services, offers a comprehensive methodology to streamline this process. In this guide, we will delve into the 12 essential steps recommended by Kodexo Labs for training stable diffusion models effectively, ensuring both efficiency and high-quality results.
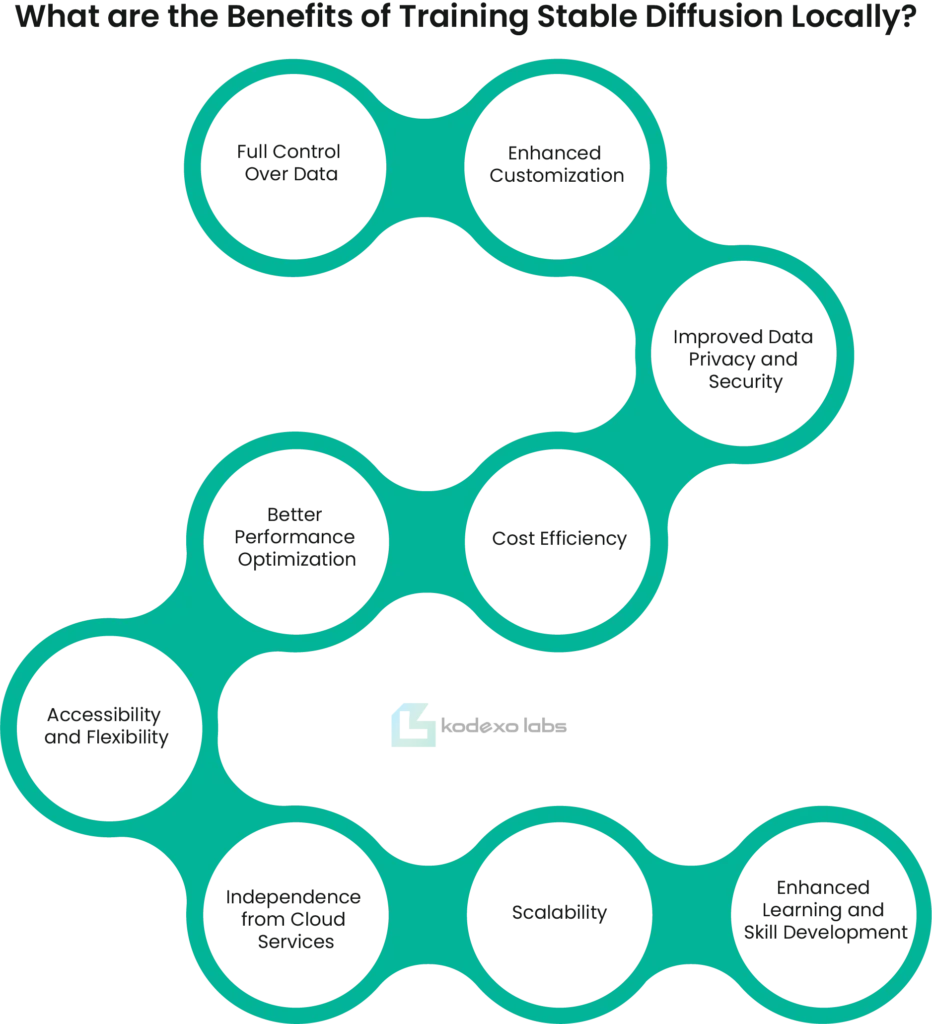
Step 1: Define Objectives and Requirements
Begin by clearly outlining the objectives and requirements of the stable diffusion model. Understanding the end goals, such as the specific types of data it will handle and the expected performance metrics, sets a solid foundation for the training stable diffusion model.
Step 2: Data Collection and Preparation
Collect a substantial and diverse dataset relevant to your application. Data preparation includes cleaning, normalization, and augmentation to enhance the dataset’s quality and representativeness.
Step 3: Data Annotation
Accurate data annotation is crucial for supervised learning tasks. Utilize Kodexo Labs’ data annotation tools or services to label the dataset precisely, ensuring that training stable diffusion model learns from correctly tagged data.
Step 4: Split Data into Training, Validation, and Test Sets
Divide the dataset into training, validation, and test sets to enable proper evaluation of the model’s performance. Typically, a split of 70% training, 20% validation, and 10% test data is recommended.
Step 5: Model Selection
Choose an appropriate model architecture for training stable diffusion from scratch. Kodexo Labs provides pre-trained models and customizable architectures that can be fine-tuned according to specific requirements.
Step 6: Setting Up the Development Environment
Establish a robust development environment using Kodexo Labs’ development services. This includes setting up necessary libraries, frameworks, and computational resources like GPUs for efficient training.
Step 7: Model Initialization and Configuration
Initialize the model with suitable parameters and configure hyperparameters such as learning rate, batch size, and epochs. Kodexo Labs’ platform offers tools to experiment with different configurations seamlessly.
Step 8: Training the Model
Commence the training process by feeding the training dataset into the model. Monitor the training progress and adjust parameters if necessary to optimize performance.
Step 9: Validation and Hyperparameter Tuning
Regularly validate the model using the validation set to assess its performance. Fine-tune hyperparameters based on validation results to improve accuracy and reduce overfitting.
Step 10: Model Evaluation
Evaluate the trained model using the test set to measure its effectiveness. Metrics such as accuracy, precision, recall, and F1-score are essential for a comprehensive evaluation.
Step 11: Model Optimization
Optimize the model for deployment by reducing its size and complexity without compromising performance. Techniques such as pruning and quantization can be employed for this purpose.
Step 12: Deployment and Monitoring
Deploy the optimized model into the production environment. Continuous monitoring and maintenance are crucial to ensure the model performs well in real-world scenarios. Kodexo Labs’ services offer monitoring tools to track the model’s performance post-deployment.
By following these 12 steps, leveraging Kodexo Labs’ development services, organizations can efficiently train and deploy stable diffusion models. This systematic approach ensures that the models are not only effective but also robust and scalable, meeting the desired objectives with precision.
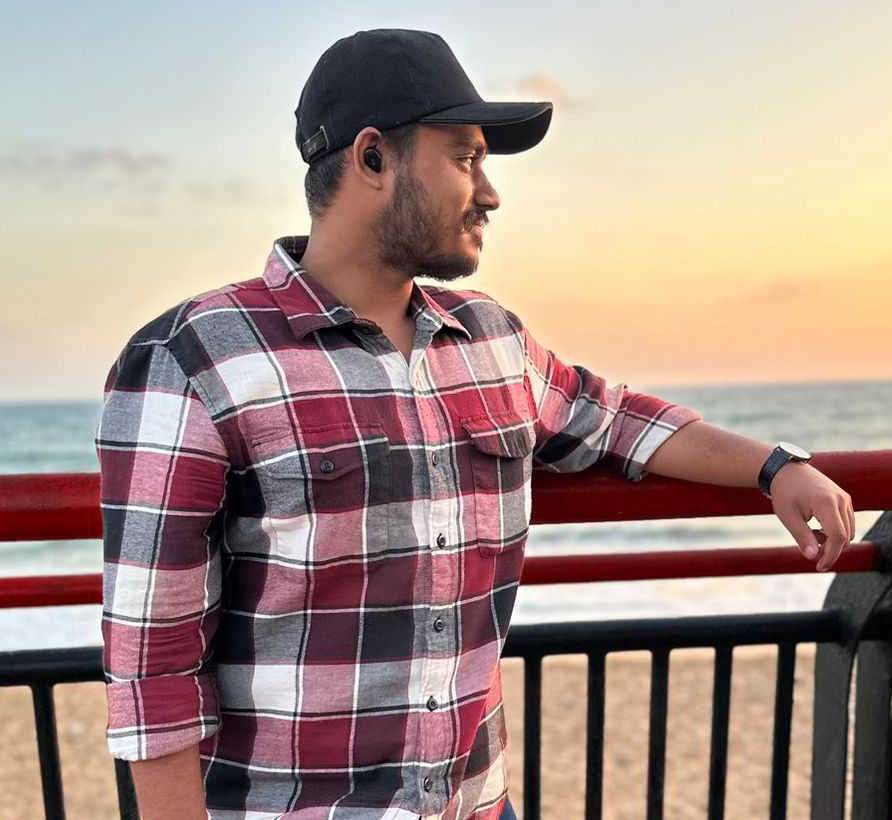
Author Bio
Syed Ali Hasan Shah, a content writer at Kodexo Labs with knowledge of data science, cloud computing, AI, machine learning, and cyber security. In an effort to increase awareness of AI’s potential, his engrossing and educational content clarifies technical challenges for a variety of audiences, especially business owners.